How to Get Started with Data Annotation: Choosing a Vendor
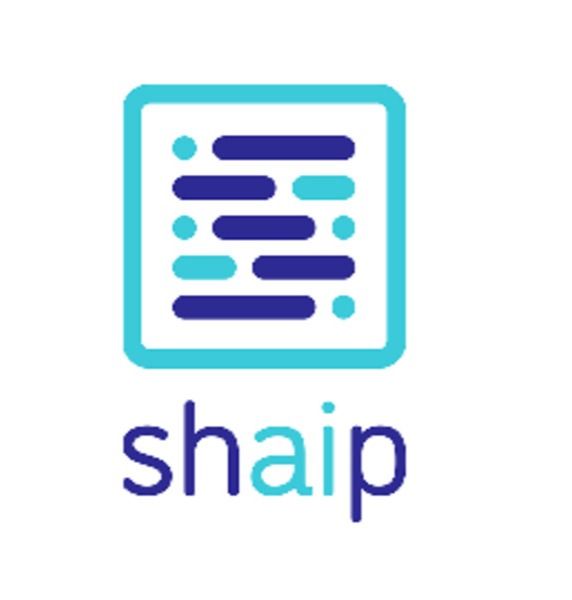
@shaipshaip
Shaip is a leader and innovator in the structured AI Data solutions category.
Artificial intelligence is getting smarter by the day. Today, powerful machine learning algorithms are within reach of normal businesses, and algorithms requiring processing power that would once have been reserved for massive mainframes can now be deployed on affordable cloud servers. Natural language processing of the kind seen in popular chatbots may appear mundane, but it wasn’t all that long ago it was the stuff of science fiction.
You Need AI in Your Business
Gartner ranks augmented data management, NLP and conversation AI as some of the key coming trends for data and analytics. Data annotation is an important part of supporting AI to perform those tasks well. If you’re not putting good data into your models, you won’t get smart responses out. According to Gartner, up to 85% of AI projects will deliver erroneous results by 2022 due to biases in their training data.
Data mining and annotation skills are essential, yet 53% of organizations say that their own data mining skills are “limited”.
What is Data Annotation?
Data annotation is a crucial part of making your AI smarter. It involves labeling the data that you feed to your machine learning algorithms so that the algorithms can learn to process the information that they see correctly. Data annotation is a painstaking process that can involve adding precise and mundane (to humans) notes to thousands upon thousands of images, pieces of text, or other data.
For data annotation to be done correctly, it is important that the humans who are working on the training data understand the scope of the project and what the algorithm is looking for. Having trained teamwork on the project, or at least explain to your in-house team what is required for data labeling, can help to maximise the efficiency of the project.
What Needs to Be Annotated?
Exactly what will need to be annotated depends on the type of project that you’re working on. A deep learning algorithm would need different inputs to a conversational AI or chatbot.
Data annotation takes time. According to a survey conducted by Algorithmia, 40% of companies report that it takes more than a month to deploy a machine learning model into production, and 81 percent of companies say that the training process is more difficult than they thought it would be.
Should you outsource or annotate in-house?
It can be tempting to handle data annotation within your business, however, this is a waste of your in-house data scientist time. Data scientists reportedly spend just 20% of their time on analysis, with the bulk of their work being sanitizing and processing data. Outsourcing your data annotation will give your project a chance to get off the ground, and free up your data scientists to focus on their core skills.
Some basic annotations can be “crowdsourced”, and this is an affordable way of getting a significant number of annotations done. If your algorithm requires more than simple sentiment data or descriptions of mundane pictures, then your annotation team may need more detailed training. Some annotations require input from subject matter experts. This is particularly true in engineering, legal, scientific or medical fields. If your machine learning algorithm is going to be creating predictions or responding in mission-critical situations it is vital that the model is given accurate inputs. Only a subject matter expert can train an AI in a complex subject.
Choosing Your Annotation Vendor
If you are looking for assistance to train your AI, consider the following:
- How much data do you need them to work through?
- How diverse a data-set do you need?
- Is the data sensitive?
- If the data is sensitive, what precautions do you want the team to take?
- How quickly do you need the annotations to be done?
- How important is accuracy?
- Do the annotators need specific knowledge?
Once you have your data annotation wishlist, you can start the process of choosing a vendor.
- Write a Statement of Work:
A statement of work defines the expectations that you have of the data annotation vendor, including the workflow, scalability requirements, delivery schedule, and quality standards. These should be clear, measurable, and agreed upon by both parties before any work begins.
- Evaluate Several Companies:Make a list of data annotation vendors. Rather than looking just at their websites, evaluate their company histories. Look for press releases, media coverage, past clients, etc., to get an idea of how established they are and the scale they work at.
- Evaluate Each Vendor’s Tools and Systems:Each vendor will most likely have its own in-house tools and systems. Ask to see examples of them in action. Do the systems make the job easy? Do they look like they should work well for large amounts of data or are they likely to be error-prone? If the vendor is crowdsourcing work, are the systems secure and does the company collect confidentiality agreements/NDAs for all of their annotators? Do you feel confident that your company’s data will be protected?
Ask each vendor what their quality control system is. How do they guarantee that the data they are processing?
- Start Small:Ask the vendors on your shortlist to commit to a small ‘proof of concept’ project. This will allow you to see some sample data, and also find out whether they are capable of delivering on time. If the sample project goes well, you can start to scale up.
For some annotations, such as sentiment data, there is an element of subjectivity, and that’s acceptable. A face that seems “very happy” to one person may be judged as just “happy” by another. That’s why having a high volume of annotations helps, since a large number of ratings helps to smooth out differences of opinion. In scientific and medical models, there is far less room for differences of opinion.
If you are considering outsourcing data annotation, talk to the team at Shaip about your project. The annotation experts have experience with many AI projects, including deep learning, chatbots, and predictive models, and can help you get your project off to the best possible start.
Author Bio

Vatsal Ghiya is a serial entrepreneur with more than 20 years of experience in healthcare AI software and services. He is a CEO and co-founder of Shaip, which enables the on-demand scaling of our platform, processes, and people for companies with the most demanding machine learning and artificial intelligence initiatives.
Tags
Create your free account to unlock your custom reading experience.